
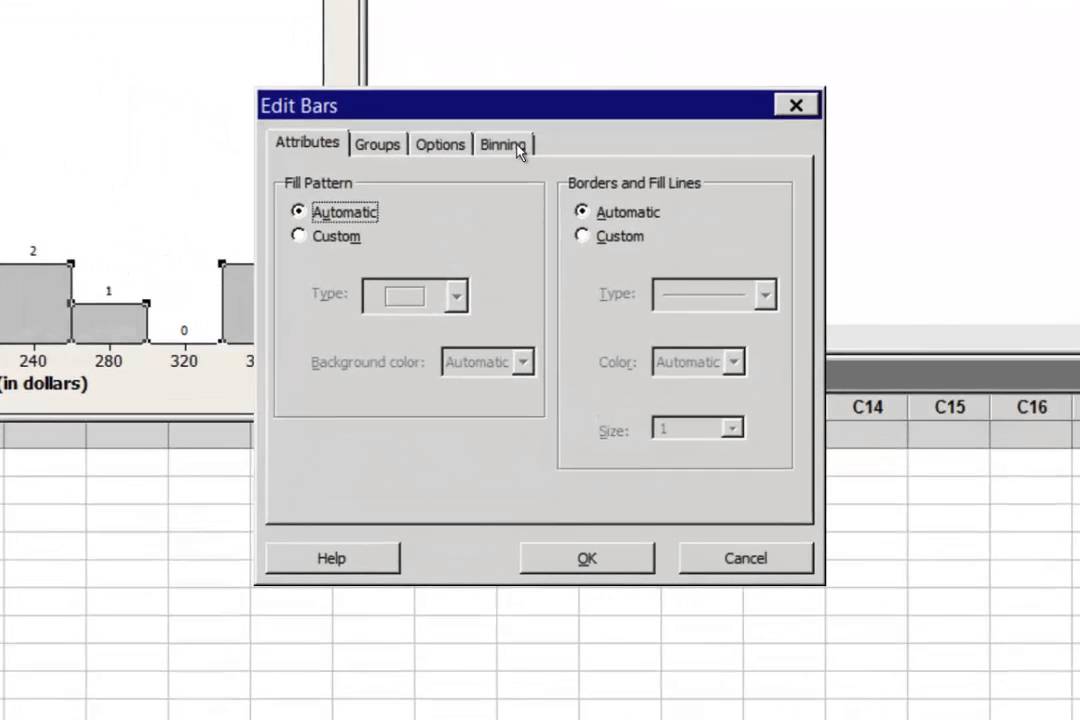
If your needs are beyond what Minitab offers, consider MATLAB, R, Python, or a combination of the three.
#MINITAB IN EXCEL FREE#
More information about the Minitab suite of products, along with a free trial, can be found on the company's website. Relying on standard programming to run predictive models may not result in the most accurate model for your organization. The key to predictive analytics is understanding the mathematics behind the model. A word of caution though, while it is wonderful that Minitab is making predictive modeling easy. But the vendor has been working on Python integration and improving its predictive modeling offerings. Descriptive statistics are where Minitab truly shines.
#MINITAB IN EXCEL SERIES#
Some of the most popular features of the platform are basic statistics, graphics, regression, statistical process control, simulations, and time series and forecasting.
#MINITAB IN EXCEL SOFTWARE#
The types of data the software works best with are numerical, text, and date-time data types. This software is quite common, especially with engineering related fields, because its dropdown interface lessens the learning curve for users of all backgrounds, and its quality improvement features align perfectly with Lean and Six Sigma methods. Minitab essentially takes the functionalities of Excel to another level, making heavy statistics easy to grasp for all users.
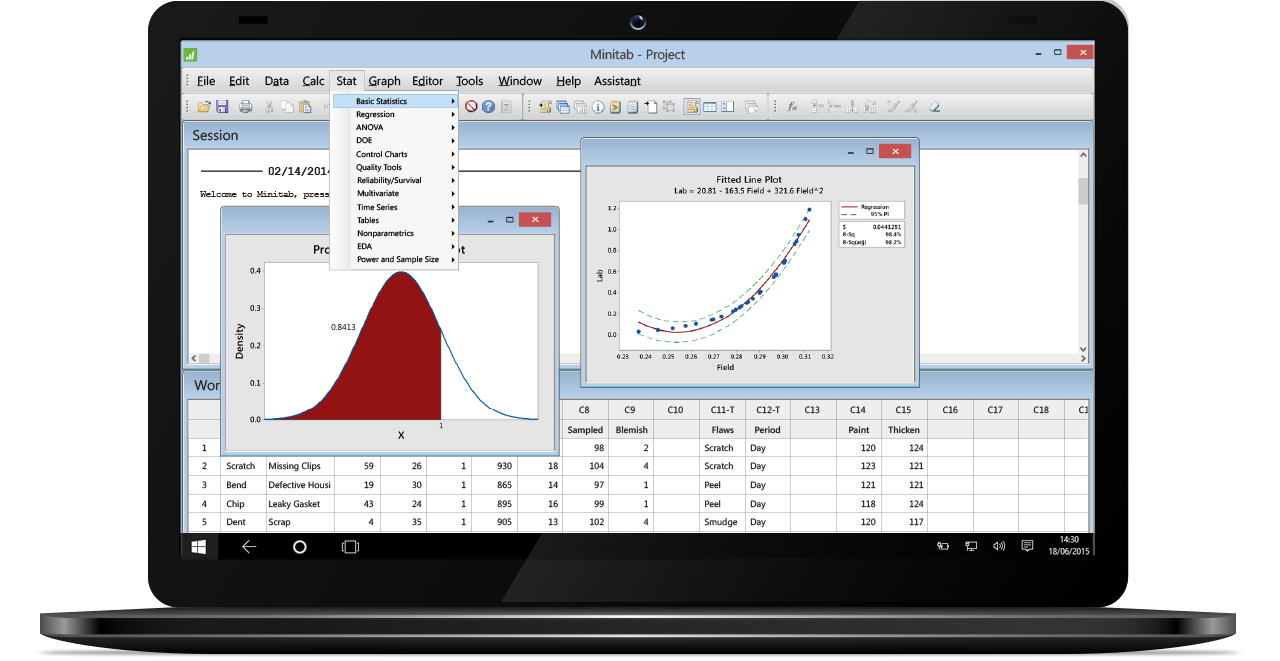
Each of these options have their own strengths and weaknesses which are important to note when determining which is right for your organization. If Excel cannot meet the needs of the analysis, There are several other options including, but not limited to, Minitab, MATLAB, R, and Python. That makes it less appropriate for deep analytics. In particular, Excel cannot store large quantities of data. However, there are some limitations to Excel. Using Excel is fairly straight forward because in a typical use case, the dataset and analysis are housed in the same file, and the formulas are thoroughly explained. Excel is widely used across all industries and for good reason, it is an incredibly powerful, easy to use software, and there are a ton of great resources online that provides solutions to almost any issue an analyst might run into. When discussing the different tools that can be used for analysis, the best place to start is with Microsoft Excel.
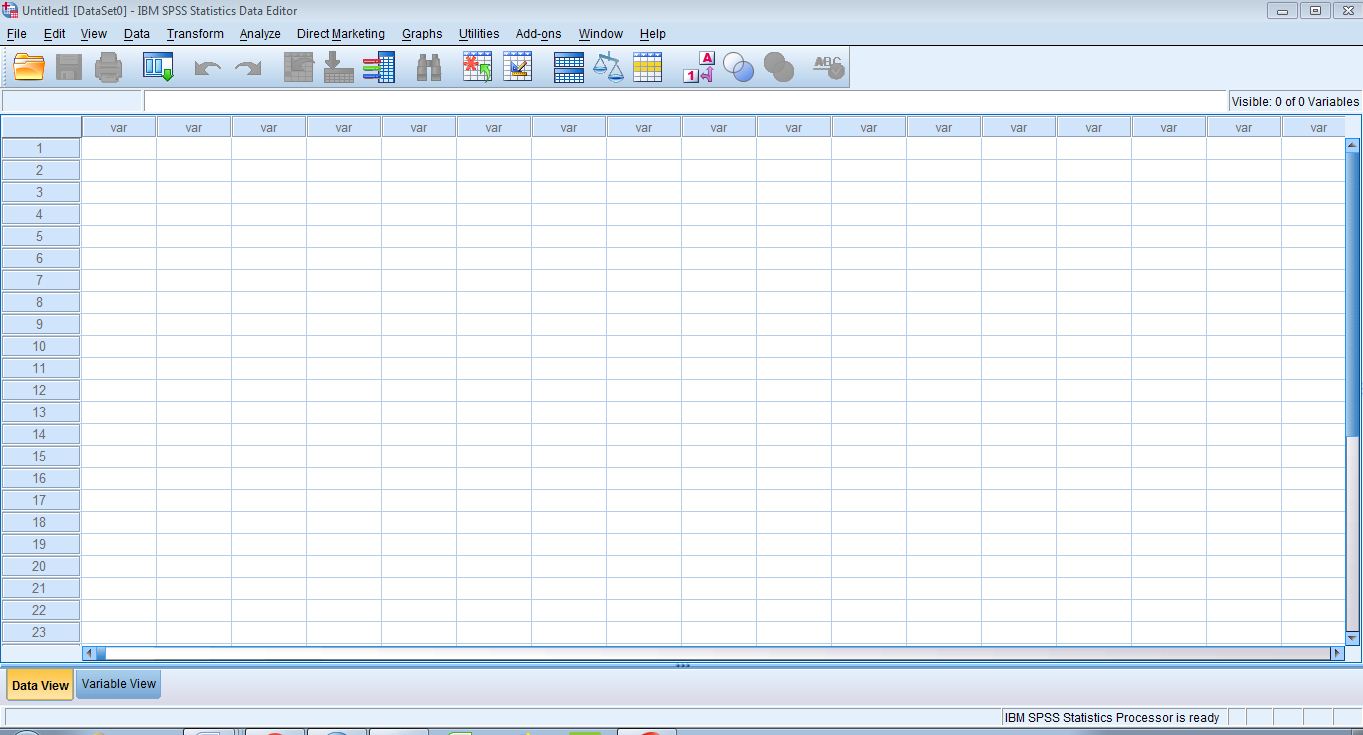
To learn more about the specialization, check out a video overview at. It is the second course in the Data-Driven Decision Making (DDDM) specialization. It is helpful if learners have some familiarity with reading reports, gathering and using data, and interpreting visualizations. This course is ideal for individuals keen on developing a data-driven mindset that derives powerful insights useful for improving a company’s bottom line. Material features online lectures, videos, demos, project work, readings and discussions. The course also addresses do’s and don’ts of presenting data visually, visualization software (Tableau, Excel, Power BI), and creating a data story. Deeper examination is spent on statistical process control (SPC), which is a method for studying variation over time. It begins with common hurdles that obstruct adoption of a data-driven culture before introducing data analysis tools (R software, Minitab, MATLAB, and Python). By the end of this course, learners are provided a high-level overview of data analysis and visualization tools, and are prepared to discuss best practices and develop an ensuing action plan that addresses key discoveries.
